판다스 데이터 프레임으로 여러가지 그래프를 그리고, Customizing 하는 방법에 대해 알아보겠다.
1. Import Library
matplotlib 와 seaborn을 활용한다.
import matplotlib.pyplot as plt
import seaborn as sns
import numpy as np
import pandas as pd
import random
2. Create Sample Data Set
50개의 random 한 데이터를 만들어 준다.
group별로 그래프를 customizing 하기 위해 A, B, C로 이루어진 'group' 컬럼도 생성한다.
data = pd.DataFrame({"x":np.random.rand(50),
"y":np.random.rand(50),
"z":np.random.rand(50),
'group': np.random.choice(['A','B','C'],50)})
3. Line Plot
가장 기본적인 Line Plot은 다음과 같이 그릴 수 있다.
x축은 data의 index, y축은 지정해 준 'x' 로 그려진다.
data['x'].plot()
'x' 뿐 아니라, 'y' 까지 함께 그려주고 싶으면 다음과 같이 해주면 된다.
같은 방법으로 'z' 까지도 그릴 수 있다.
data[['x','y']].plot(figsize = (10,5))
subplots = True 를 지정해주면 하나의 plot 이 아니라 컬럼별로 각각 그려진다.
단, figsize는 각각 하나의 size가 아니라, 전체 plot의 사이즈임을 주의한다.
data[['x','y']].plot(figsize = (10,10), # 전체 figure size
subplots = True)
선 색상을 바꾸거나, 그래프의 제목을 지정하는 등 Cutomizing 하는 방법은 다음과 같다.
figsize 로 그래프 전체 사이즈를 지정하고,
color 로 선 색상을 지정하며,
xlabel, ylabel 로 x축과 y축의 이름을 지정한다.
xlim, ylim으로 x축, y축의 범위를 지정하고,
title로 그래프의 제목을 지정한다.
data['x'].plot(figsize = (10,5), # figure size(width, height)
color='r', # color
xlabel="index", # label of x
ylabel = 'X', # label of y
xlim=(-5,55), # x축 범위
title = "Plot of X") # title
수평선은 axhline()으로 그린다.
수평선을 추가할 y값을 넣어주고,
color로 색상을 지정하고,
linestyle, linewidth 등으로 Customizing 해준다.
fig, ax = plt.subplots()
data['x'].plot(ax = ax,
figsize = (10,5), # figure size(width, height)
color='gray', # color
xlabel="index", # label of x
ylabel = 'X', # label of y
xlim=(-5,55), # x축 범위
title = "Plot of X") # title
plt.axhline(data.x.mean(), # y축 위치
color='r', # 색상
linestyle='--', # dashed lind
linewidth='2') # size
x축 전체가 아닌, 원하는 만큼만도 그릴 수 있다.
axhline()이 아닌 hlines()를 활용하며, xmin, xmax로 원하는 x 축의 범위를 넣어준다.
(axhline() 도 xmin, xmax로 입력 가능하나, 실제값이 아니라 0~1 사이의 상대값으로 넣어야 한다.)
fig, ax = plt.subplots()
data['x'].plot(ax = ax,
figsize = (10,5), # figure size(width, height)
color='gray', # color
xlabel="index", # label of x
ylabel = 'X', # label of y
xlim=(-5,55), # x축 범위
title = "Plot of X") # title
plt.hlines(data.x.mean(), # y축 위치
xmin = 10,
xmax = 30,
color='r', # 색상
linestyle='--') # size
자주 사용되는 라인스타일은 다음과 같다.
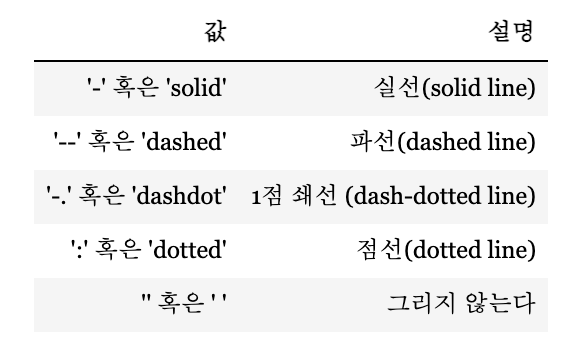
수직선은 axvline() or vlines() 로 그릴 수 있다.
fig, ax = plt.subplots()
data['x'].plot(ax = ax,
figsize = (10,5), # figure size(width, height)
color='gray', # color
xlabel="index", # label of x
ylabel = 'X', # label of y
xlim=(-5,55), # x축 범위
title = "Plot of X") # title
plt.axvline(30, # x축 위치
color='r', # 색상
linestyle=':', # dashed lind
linewidth='2') # size
4. Scatter Plot
scatter plot은 위 기본 line plot에 kind="scatter" 를 추가해주면 된다.
단, 꼭 x와 y를 지정해 주어야 한다.
data.plot(kind = "scatter",
x = 'x',
y = 'y',
figsize = (10,5))
이를 group별로 다른 색을 지정해서 그리는 방법은 다음과 같다.
먼저 plt.subplots() 로 도화지를 펴주고,
각 group별로 group.plot() 으로 도화지에 그림을 그려준다.
각각 color와 label을 지정해주어 group별로 다른 색상으로 그려준다.
fig, ax = plt.subplots(figsize = (10,5)) # fig size
colors = ['r','g','b'] # group별 color 지정
for i, (name,group) in enumerate(data.groupby('group')):
group.plot(ax=ax,
kind='scatter',
x="x", y="y",
s=50, # size of point
color=colors[i],
label = name)
참고로 Figure, Axes, Axis의 개념은 다음과 같다.
Figure는 그래프 전체, Axes는 실제 그림이 그려지는 부분, Axis는 축이다.
왼쪽의 Axes는 하나이고, 오른쪽의 Axes는 두 개이다.
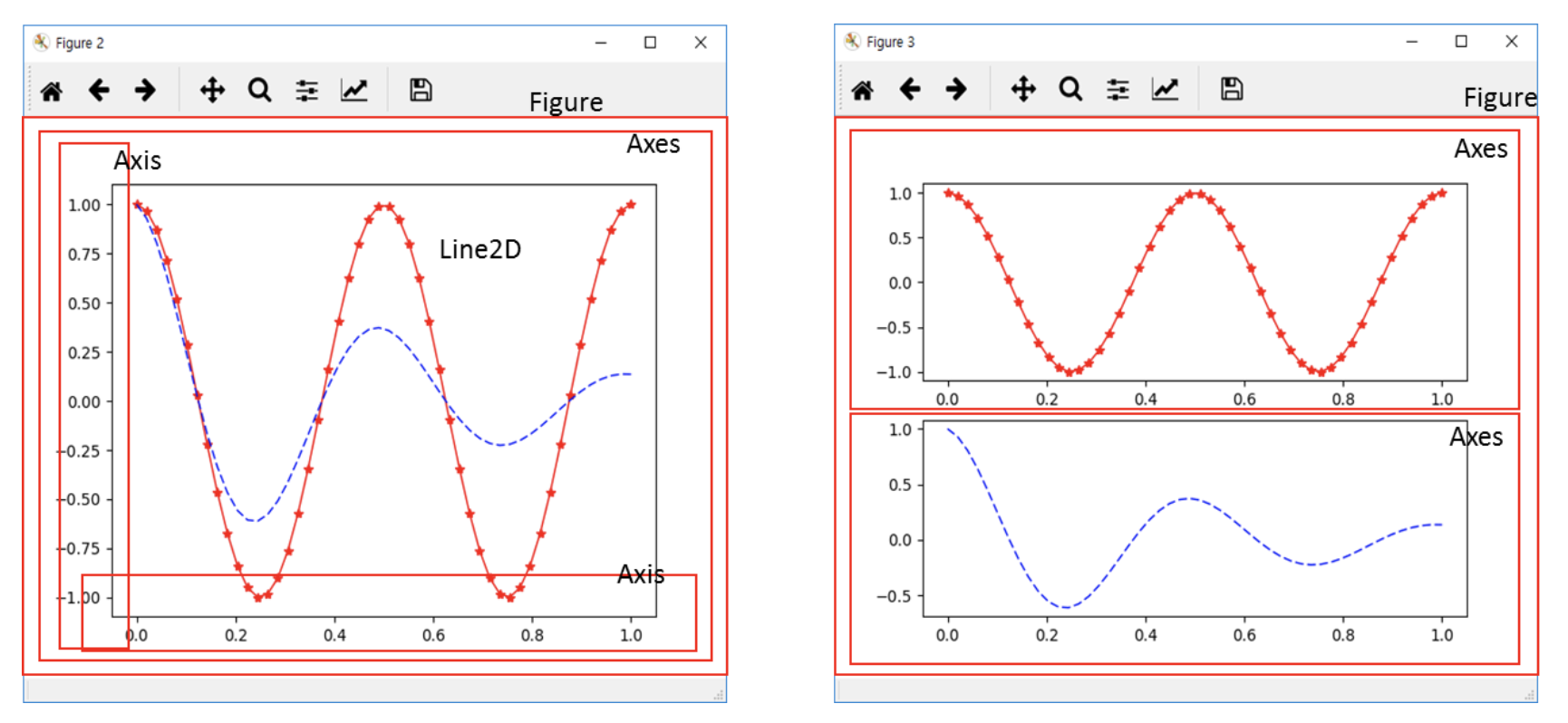
5. Box Plot
box plot은 kind = "box" 로 그려준다.
data[['x']].plot(kind="box",
figsize = (7,5))
box plot은 다음과 같이 customizing 해준다.
fig, ax = plt.subplots(figsize = (7,5)) # size
bp = ax.boxplot(data.x, patch_artist=True) # boxplot
for box in bp['boxes']: # box colors
box.set(color='#F8766D', # box line color
facecolor='Pink')
for whisker in bp['whiskers']:
whisker.set(color="DarkOrange")
for cap in bp['caps']:
cap.set(color="Gray")
for median in bp['medians']:
median.set(color="white")
각각의 색상이 의미하는 것은 다음과 같다.
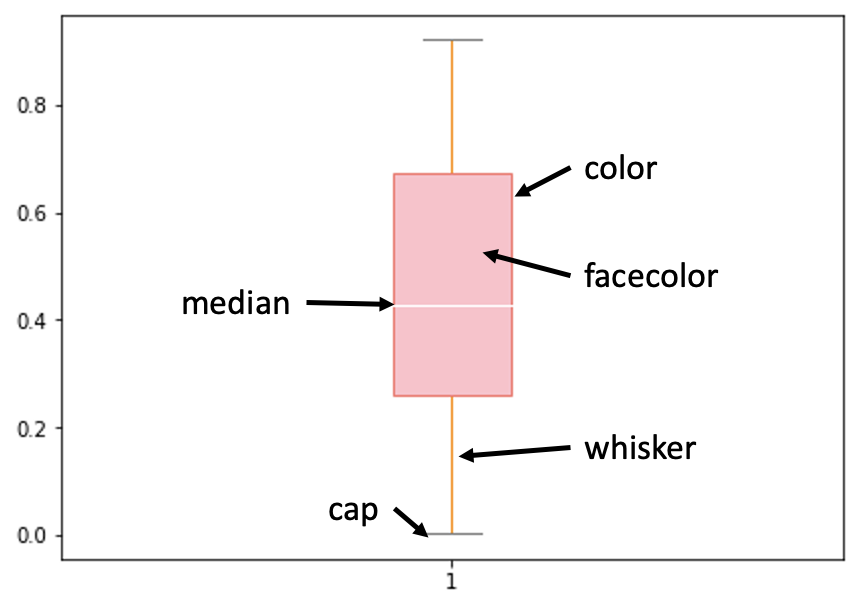
Group별로 Customizing하는 방법은 다음과 같다.
group별로 box plot을 그려주고, for문을 통해 각각 customizing한다.
fig,ax = plt.subplots(figsize=(10,5)) # size
colors = ['#F8766D',"#00BFC4","#7CAE00"] # color
bp_dict = data[['x','group']].boxplot( # drawing boxplot by group
ax = ax,
by="group",
return_type='both',
patch_artist = True,
)
plt.suptitle("")
for row_key, (ax_,row) in bp_dict.iteritems(): # set colors
ax_.set_xlabel('')
for i,box in enumerate(row['boxes']):
box.set_facecolor(colors[i])
6. Histogram
histogram은 hist() 로 그려준다.
data[['x']].hist(figsize = (10,5))
bins는 몇 개의 영역으로 쪼갤 지를 의미하고, density = True는 y 축을 밀도함수로 바꿔주어, 전체 면적 합이 1이되도록 한다.
histtype을 step으로 해주면 아래 색상이 채워지지 않는다.
data[['x']].hist(figsize = (10,5), # size
bins = 20, # 몇 개의 영역으로 쪼갤지
color = '#F8766D', # color
# density = True, 밀도함수 : 면적 합이 1
histtype = "step")
수치형 컬럼이 여러개로 이루어진 data에 바로 hist()를 그리면, 각각의 histogram이 그려진다.
layout을 통해 원하는 row 개수와 col 개수를 지정할 수 있다.
data.hist(figsize = (10,10), # size
bins = 20,
layout = (3,1),
color = "#F8766D")
7. Density Plot
density plot은 seaborn 기반으로 그려보겠다.
hist 는 histogram을 그릴 것인지, kde는 density line을 그릴 것인지이다.
density plot만 그리고 싶다면 hist는 False로, kde는 True로 그린다.
plt.figure(figsize = (18,5))
sns.distplot(data['x'],
hist = False, # histogram
kde = True, # density line
kde_kws = {'shade': True, 'linewidth': 3})
Group별로 그리는 방법은 다음과 같다.
plt.figure(figsize = (18,5))
for a in set(data.group):
subset = data[data['group'] == a]
# Draw the density plot
sns.distplot(subset['x'], hist = False, kde = True,
kde_kws = {'shade': True, 'linewidth': 3},
label = a)
plt.legend(prop={'size': 16}, title = 'label')
8. Bar Plot
group별 데이터 개수를 bar plot으로 그리면 다음과 같다.
data.value_counts('group').plot(kind="bar", figsize = (10,5))
참고로 data.value_counts('group') 의 type은 pandas Series 이다.
9. Pie Chart
역시 group별 데이터 개수로 Pie 차트를 그리면 다음과 같다.
data.value_counts('group').plot.pie(figsize = (5,5))
label과 색상 등은 다음과 같이 바꿀 수 있다.
autopct로 값을 text로 넣어줄 수도 있다. (합을 100으로 만들어준다.)
data.value_counts('group').plot.pie(
labels=["AA", "BB", "CC"], # label
colors=['#F8766D',"#00BFC4","#7CAE00"], # color
autopct="%.2f", # 소수점
fontsize=20,
figsize=(6, 6))
'Python > Plot' 카테고리의 다른 글
[Python] matplotlib 으로 pandas data 그래프 그리기 :: multiple plots, Customizing Figure Layouts, scatter, boxplot (0) | 2021.06.10 |
---|